Artificial Intelligence
on
Skin care
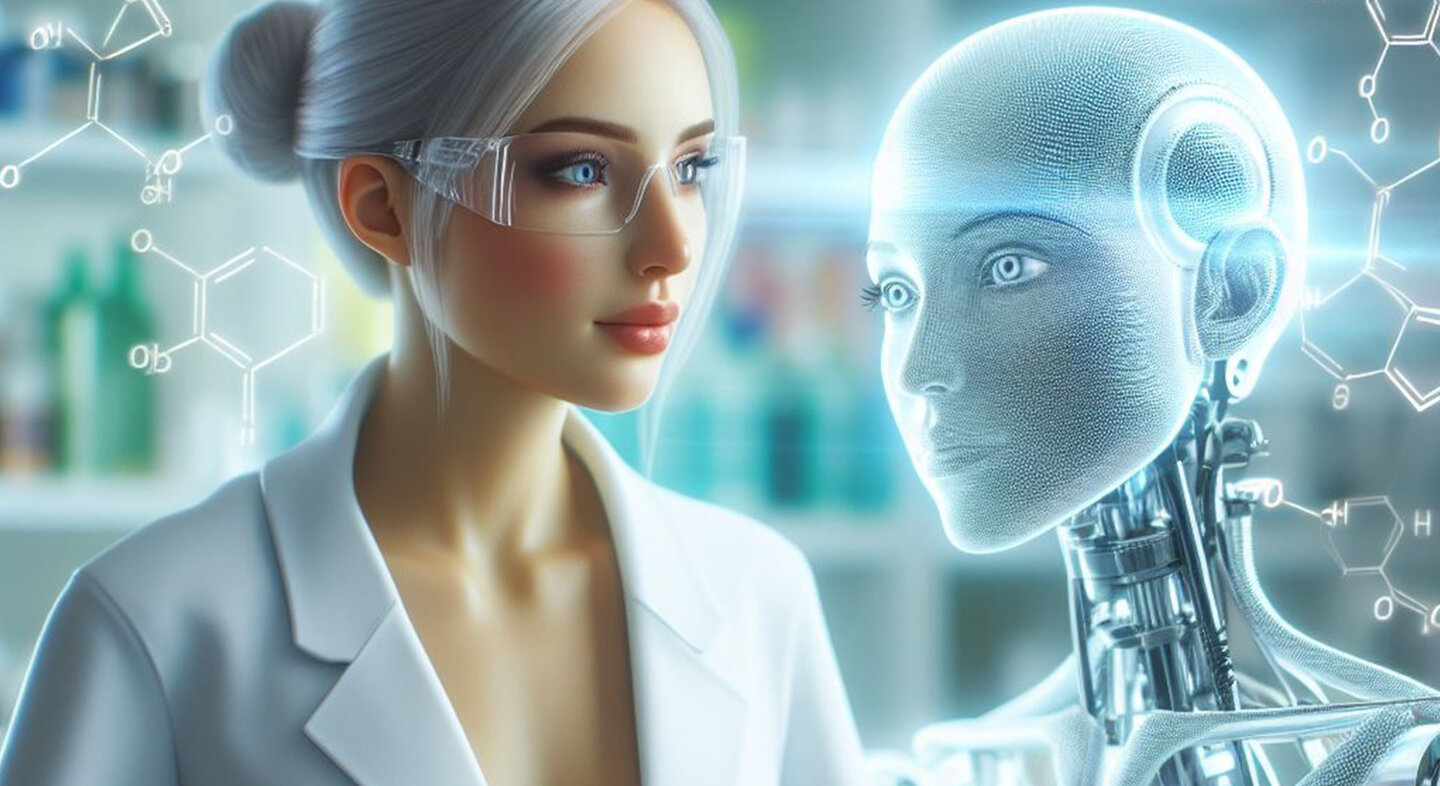
peer-reviewed
AI and surfactants
NEIL BURNS
Managing Partner Neil A Burns LLC
??????????????????
Introduction
AI (Artificial Intelligence) as most readers will know is affecting many areas of our daily lives. Since the launch of Open AI’s Chat GPT, the prevalence of AI has become more apparent to the general public. In this paper, I will use the abbreviation, AI to apply to any or all of Artificial Intelligence (AI), Machine Learning (ML) and Self-Driving Labs (SDL). We will see that, although published accounts of the use of AI in the surfactants industry are few, there are plenty of examples of AI use in the field of chemistry. These well documented industrial and academic accounts show that the field of surfactants is particularly ripe for the application of AI in three key areas to solve problems related to performance, cost and sustainability. These three areas are:
- Product Development: That is the development and synthesis of new surfactant molecules.
- Manufacturing: New or optimized process technology to make either new or existing surfactants.
- Application: The development or optimization of formulations that enable surfactants to perform their role of cleaning, emulsification, separation etc. in consumer or industrial products.
This article is not intended to be a comprehensive review of the state of the art, but provides examples of how AI can improve surfactant development, manufacturing and application and will perhaps inspire further investment and work in such areas.
“
“A study in healthy women providing probiotic yogurt for four weeks showed an improvement in emotional responses as measured by brain scans”
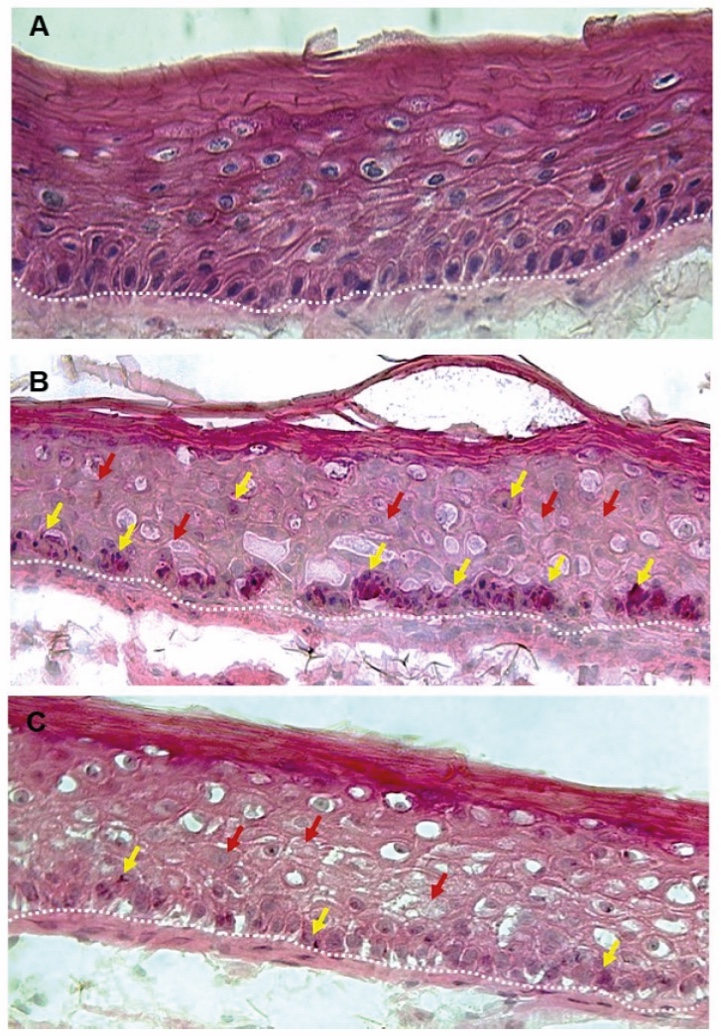
Figure 1. Skin Section with Microbiome. Most microorganisms live in the superficial layers of the stratum corneum and in the upper parts of the hair follicles. Some reside in the deeper areas of the hair follicles and are beyond the reach of ordinary disinfection procedures. There bacteria are a reservoir for recolonization after the surface bacteria are removed.
Materials and methods
Studies of major depressive disorder have been correlated with reduced Lactobacillus and Bifidobacteria and symptom severity has been correlated to changes in Firmicutes, Actinobacteria, and Bacteriodes. Gut microbiota that contain more butyrate producers have been correlated with improved quality of life (1).
A study in healthy women providing probiotic yogurt for four weeks showed an improvement in emotional responses as measured by brain scans (2). A subsequent study by Mohammadi et al. (3) investigated the impacts of probiotic yogurt and probiotic capsules over 6 weeks and found a significant improvement in depression-anxiety-stress scores in subjects taking the specific strains of probiotics contained in the yogurt or capsules. Other studies with probiotics have indicated improvements in depression scores, anxiety, postpartum depression and mood rating in an elderly population (4-7).
Other studies have indicated a benefit of probiotic supplementation in alleviating symptoms of stress. In particular, researchers have looked at stress in students as they prepared for exams, while also evaluating other health indicators such as flu and cold symptoms (1). In healthy people, there is an indication that probiotic supplementation may help to maintain memory function under conditions of acute stress.
Current Industrial Activity
Substantial investment and progress has already been made in the direct application of AI in businesses using surfactants. Unilever has been particularly active across the whole value chain, as the following accounts, published the company, show.
- Unilever is using AI to optimise its portfolio of brands and products, including surfactants, by using a data-driven tool that recommends which Stock Keeping Unit (SKUs) to delist, watch, protect or grow, based on the benefit to customers, consumers and Unilever (1). This helps to reduce waste, improve productivity and focus on core and innovative SKUs that meet consumer needs and preferences.
- Unilever is using AI to innovate by discovering new ingredients and new science, such as plant-based, sustainable palm oil alternatives for surfactants, by designing and simulating products and processes virtually, such as the world's first green carbon detergent, and by deploying products more efficiently and effectively, such as using digital twins and smart sensors to monitor and optimise production (2).
- Hindustan Lever in India uses AI to improve its supply chain resilience and agility, by using data analytics and machine learning to forecast demand, optimise inventory, reduce costs and enhance customer service (3). This helps to ensure the availability and quality of surfactants and other products, especially during times of disruption and uncertainty.
- Unilever in the UK is using AI to enhance its talent acquisition and retention, by using an artificial intelligence system to assess job interviews, reducing the time and cost of hiring and improving the diversity and fit of candidates (4).
- The company is using AI to improve its products and consumer experience, by using molecular models to improve its product formulas, faster and more effectively than in a physical lab (5), by using data to identify and predict consumer trends and preferences, such as using voice assistants and chatbots to interact with consumers. This helps to create value and impact for society and the environment, as well as for Unilever and its customers.
Surfactant Applications
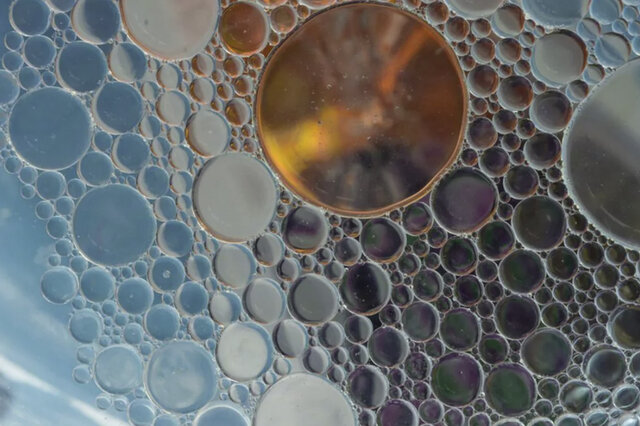
The application area lends itself particularly well to the use of AI. Active today in this area is the US company Potion AI (6). The company provides AI-powered formulation tools for beauty and personal care R&D. Their offerings include Potion GPT, next generation ingredient and formula databases and AI document processing. Potion’s work could have a significant impact on the entire surfactant value chain, from raw material suppliers to end consumers. By using their GPT technology, they can help target work toward novel surfactant molecules that have optimal properties for specific applications. By using their ingredient and formula databases, they can access and analyze a vast amount of data on surfactant performance, safety, and sustainability. By using their AI document processing, they can extract and organize relevant information from patents, scientific papers, and regulatory documents. These capabilities could enable Potion AI's customers to design and optimize surfactant formulations that are more effective, eco-friendly, and cost-efficient. A particularly interesting application for this type of capability is deformulation.
Deformulation is the process of reverse engineering a product's formulation by identifying and quantifying its ingredients. Deformulation can be used for various purposes, such as quality control, competitive analysis, patent infringement, or product improvement. However, deformulation can be challenging, time-consuming, and costly, as it requires sophisticated analytical techniques, expert knowledge, and access to large databases of ingredients and formulas.
AI can potentially enhance and simplify the deformulation process by using data-driven methods to infer the composition and structure of a product from its properties and performance. For example, AI can use machine learning to learn the relationships between ingredients and their effects on the product's characteristics, such as color, texture, fragrance, stability, or efficacy. AI can also use natural language processing to extract and analyze information from various sources, such as labels, patents, literature, or online reviews, to identify the possible ingredients and their concentrations in a product.
Surfactant Development and Synthesis
In the area of surfactant synthesis, there is surprisingly little fully documented AI work. However, there is plenty of work in the field of chemical synthesis in general. Professor Alan Aspuru-Guzik of the University of Toronto co-authored a paper, published at the end of October in the journal Autonomous Robots (7). In summary: The paper proposes an approach to automate chemistry experiments using robots by translating natural language instructions into robot-executable plans, using large language models together with task and motion planning. So you can tell your chemist robot - “make me a methyl ester of that acid” and off it goes, no further instruction needed. Adding natural language interfaces to autonomous chemistry experiment systems lowers the barrier to using complicated robotics systems and increases utility for non-expert users. However, translating natural language experiment descriptions from users into low-level robotics languages is not that easy. Furthermore, while recent advances have used large language models to generate task plans, reliably executing those plans in the real world ie. by a robot in a lab, remains challenging. To enable autonomous chemistry experiments and alleviate the workload of chemists, robots must interpret natural language commands, perceive the workspace, autonomously plan multi-step actions and motions, consider safety precautions, and interact with various laboratory equipment. The approach in the paper, CLAIRify, combines automatic iterative prompting with program verification to ensure syntactically valid programs in a data-scarce domain-specific language that incorporates environmental constraints. The generated plan is executed through solving a constrained task and motion planning problem using PDDLStream solvers to prevent spillages of liquids as well as collisions in chemistry labs (kind of a minimum requirement before even making any product). The effectiveness of the approach is demonstrated in planning chemistry experiments, with plans successfully executed on a real robot using a repertoire of robot skills and lab tools. This demo includes fundamental chemical experiments for materials synthesis: solubility and recrystallization. Further details about CLAIRify can be found at (8). It goes without saying that the methodologies and tools referenced here could have a big impact on surfactant synthesis work.
Surfactant Process Technology
In the area of process technology for surfactants, there is similarly little fully documented work specifically concerning surfactants. However, applications of AI in the field of chemical engineering are widely reported and have obvious implications for surfactant process technology. A good example is a joint project between MIT and the University of Ghent which was written up in the journal, Frontiers in Chemical Engineering (9). The paper, entitled Artificial Intelligence for Computer Aided Synthesis in Flow, takes a machine learning approach to figuring out whether and how a particular reaction may benefit from being done in a flow system. The surfactant industry has a history of certain reactions being transitioned from batch to continuous flow, particularly sulfonation and sulfation used in the production of Linear Alkylbenzene Sulfonates, Alcohol Sulfates, Alcohol Ether Sulfates and Alpha Olefin Sulfonates. This MIT/Ghent project therefore could be of particular interest to companies considering flow chemistry for other surfactant-relevant reactions, such as alkoxylation.
As the paper explains, running a process in a continuous fashion introduces many additional considerations for process design. Reactions in which some reagents are solids or those in which the equilibrium is driven in a certain direction via precipitation of the products are difficult to develop in flow as the solids can clog the channels. Improper combinations of solvents and reactants, products, or catalysts can also result in precipitation of certain compounds, again increasing the risk of clogging. Reactions requiring long residence times may require impractically long reactors or inefficiently low flow rates. At very low flow rates, mixing becomes an important issue, implying that the use of a stirred spheroidal reactor is much more economical. As a result, it is very difficult to anticipate whether a synthesis can be performed continuously in an economic way. The MIT/Ghent project used a data-driven method, based on a statistical analysis of published reactions, that can identify such continuous syntheses and can potentially guide retrosynthetic software toward such syntheses.
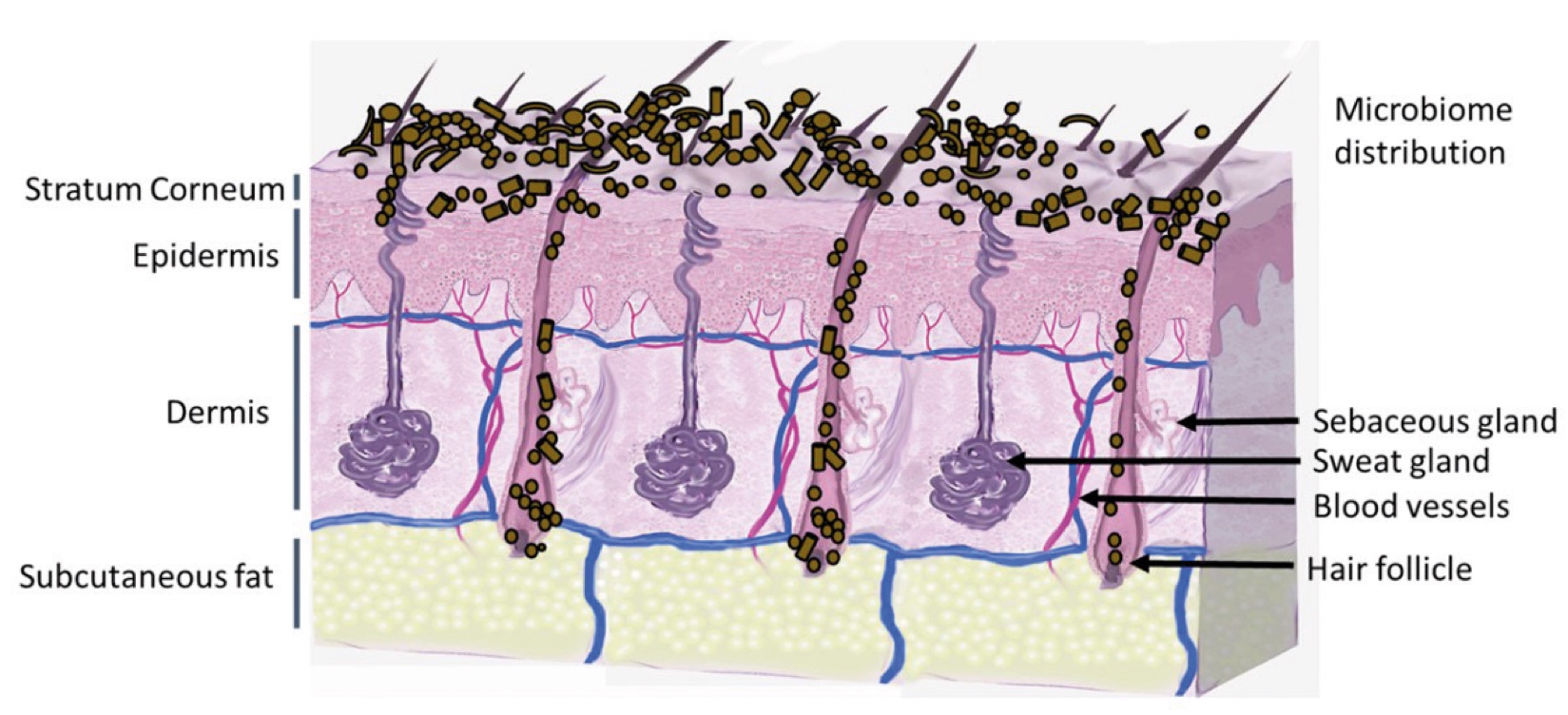
Figure 2. Skin Section with Microbiome. Most microorganisms live in the superficial layers of the stratum corneum and in the upper parts of the hair follicles. Some reside in the deeper areas of the hair follicles and are beyond the reach of ordinary disinfection procedures. There bacteria are a reservoir for recolonization after the surface bacteria are removed.
Conclusions
Having spent 40 years in the chemical industry, I believe now, more firmly than ever, that good chemistry takes time, money, trial and error - and much manual labor in the lab, pilot plant and plant. The application of AI is already demonstrating that more and better chemistry can be done more quickly and cost-effectively. The impact on the surfactant value is just beginning to be felt and there is obviously a lot of potential benefit here to be realized.
References and notes
- Using AI to optimise our portfolio | Unilever
- How AI and digital help us innovate faster and smarter | Unilever
- HUL leverages AI, ML, data for a smarter supply chain
- Unilever saves on recruiters by using AI to assess job interviews
- Unilever uses AI to improve products - Retail Brew
- Potion
- https://link.springer.com/article/10.1007/s10514-023-10136-2
- https://ac-rad.github.io/clairify/
- https://www.frontiersin.org/articles/10.3389/fceng.2020.00005/full